In this post, we will see briefly how to perform downstream analysis for RNA-seq data.
The only difference is that we can run all downstream analyses on the raw read count matrix, or the UMI-deduplicated count matrix.
Standard analysis pipelines are scripted in R or in Python. In this post we will mainly use R because it’s the accepted standard for RNA-seq analyses, but similar analyses and results can be obtained using Python. First, the data needs to be loaded from the file into the R environment, using base commands such as read.table, or more advanced (faster) ones, such as fread (from the data.table package).
# Reading the transcriptomics data
library(data.table)
data.raw <- fread("my_count_matrix.txt", sep="\t", header = T, data.table = F)
rownames(data.raw) <- data.raw[,1]
data.raw <- data.raw[,-1]
Of note, here we use the tabulation as separator, and suppose that the count matrix has a header (with the sample names), and that the first column contains gene names (or identifiers).
Finally, we remove the first column containing the gene names. Of course, this is to be repeated if you need to load external metadata, for example for loading groups, covariates, or other phenotypes.
# Compute depth per sample
data.meta <- data.frame(samples =ccolnames(data.raw), depth = colSums(data.raw))
# Filter samples that did not aggregate at least 1M reads
outliers <- subset(data.meta, depth < 1E6)$samples
data.raw <- data.raw[,-which(colnames(data.raw) %in% outliers)]
# Filter low expressed genes
data.raw <- data.raw[rowSums(data.raw > 1) > 4,] # At least 1 read in 4 samples
Once the data is filtered, it should be normalized. In particular to remove the effect of sequencing depth and thus be able to compare the samples on a similar depth basis.
# Normalization using limma-voom
library(limma)
data.norm <- voom(counts = data.raw, normalize.method="quantile")$E
Then, at this stage, we usually want to visualize our data. We can do this using dimension reduction methods such as Principal Component Analysis (PCA) or MultiDimensional Scaling (MDS).
# PCA
data.pca <- data.frame(prcomp(t(data.norm), center = T, scale. = T)$x)
# Plot
library(ggplot2)
ggplot(data.pca, aes(x = PC1, y = PC2)) + geom_point()
.jpg?width=906&name=4061ce_dd02d1ff48f84dad9fd30f3e0e21673b_mv2%20(1).jpg)
# Importing the biological effect studied (from a metadata file, or manually, or…)
data.condition <- c(T, T, T, T, …, F, F, F, F) # Binary condition here
# Plotting the PCA with coloring by condition
data.pca$condition <- data.condition
ggplot(data.pca, aes(x = PC1, y = PC2, col = condition)) + geom_point()
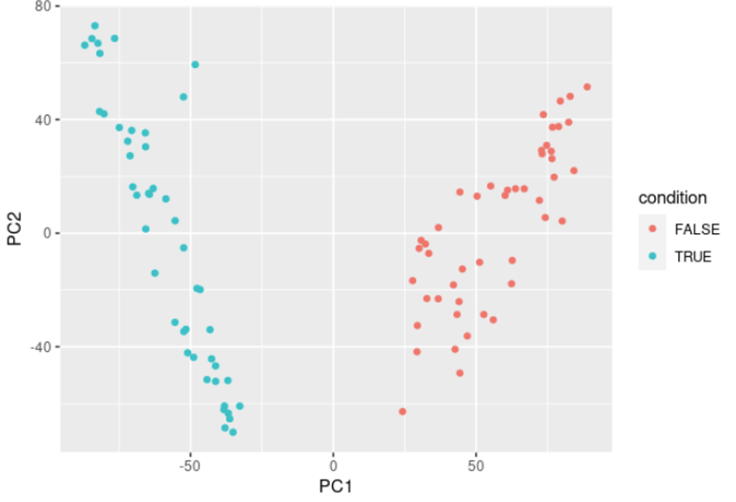
# Remove genes without variance
data.norm <- data.frame(data.norm[which(apply(data.norm, 1, var) != 0),])
# Create the design matrix based on your condition
# Of note : Here you can add covariates to the model (batch, sex, …) if you have in your data that may bias the signal of your biological condition
data.design <- model.matrix(~data.condition)
# Run the DE model
fit <- lmFit(data.norm, data.design)
fit <- eBayes(fit)
# Create the table with all DE results
DE.table <- topTable(fit, n=Inf, adjust="fdr", sort.by = "none")
# Volcano plot
ggplot(DE, aes(x = logFC, y = -log10(P.Value))) + geom_point()
This will generate the following plot:
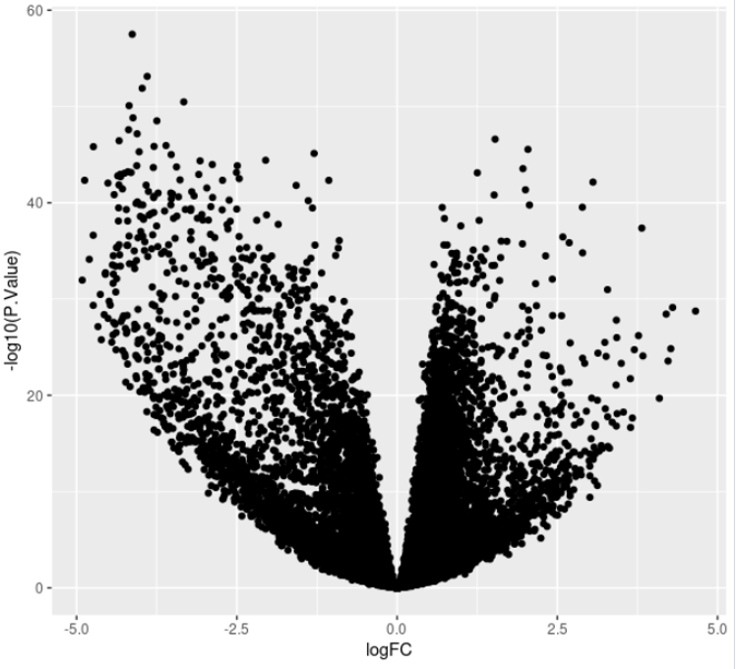
# Filter results (Here FDR <= 5% and FC > 2)
DE$is.DE <- DE$adj.P.Val <= 0.05 & abs(DE$logFC) > log2(2)
# Volcano plot
ggplot(DE, aes(x = logFC, y = -log10(P.Value), col = is.DE)) + geom_point()
# Filter the DE table
DE <- subset(DE, is.DE)
message(nrow(DE), " DE genes found: ", sum(DE$logFC > 0), " up-regulated, and ", sum(DE$logFC < 0), " down-regulated")
Which messages something like: "3344 DE genes found: 1326 up-regulated, and 2018 down-regulated" and plots the following:
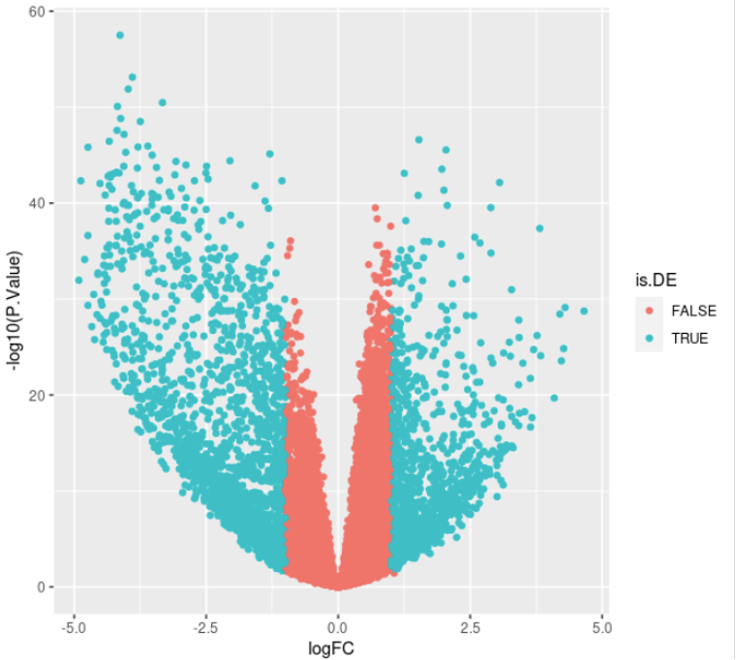
# Sort the DE genes by effect size (Fold Change)
# Top up-regulated
DE <- DE[with(DE, order(-logFC)),]
top_20_up_genes <- rownames(DE)[1:20] # Keeping the top up-regulated genes for later
fwrite(x = DE, file = "DE.genes.txt", sep = "\t", quote = F, row.names = T, col.names = T)
# Or alternatively, sort by putting the down-regulated genes at the top
DE <- DE[with(DE, order(logFC)),]
top_20_down_genes <- rownames(DE)[1:20] # Keeping the top 20 down-regulated genes
You can then sort the DE table and save it as an external text file using write.table or fwrite
# Create a data.frame containing the condition, for annotation of the heatmap
data.annotation <- data.frame(row.names = colnames(data.norm), condition = factor(data.condition))
# Plot the heatmap
library(pheatmap)
pheatmap(data.norm[c(top_20_up_genes,top_20_down_genes),], scale = "row", annotation_col = data.annotation)
This will generate the following plot:
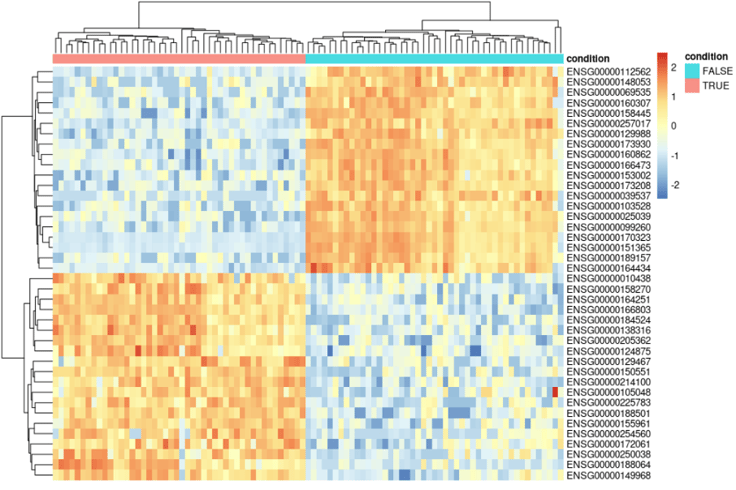
For more information about RNA-seq and BRB-seq data analysis do not hesitate to reach out at info@alitheagenomics.com