The largest blood cell genotyping and transcriptomic study ever performed has shown that a much higher number of common changes in the DNA sequence affect gene expression in blood cells than previously thought (Võsa et al. 2021). In this article, we look at how the use of RNA-seq in this study contributed to these exciting new discoveries in the genetic regulation of blood cells.
Summary:
To detect eQTLs in blood cells, the eQTLGen Consortium analyzed a data set comprising high-throughput genomic and transcriptomic technologies, such as whole genome sequencing, RNA-seq and DNA variant and expression microarrays (Fig. 1) (Võsa et al. 2021). It included 37 studies with more than 31,000 study participants (Võsa et al. 2021).
Thanks to these high-throughput technologies including RNA-seq, and the large sample size, researchers discovered that genetic variants in close proximity to genes (cis-eQTLs) regulate 88% of all genes analyzed. This often includes a physical interaction between the variant and the gene (Võsa et al. 2021). In contrast, genomic variants over 5Mb away from genes (trans-eQTLs) regulate one third of all genes analyzed, likely due to transcription factor activity (Võsa et al. 2021). For example, one genetic variant possibly regulates the neuronal repressor REST and the expression of 88 neuronal genes in trans as a result (Võsa et al. 2021).
How RNA-seq was used:
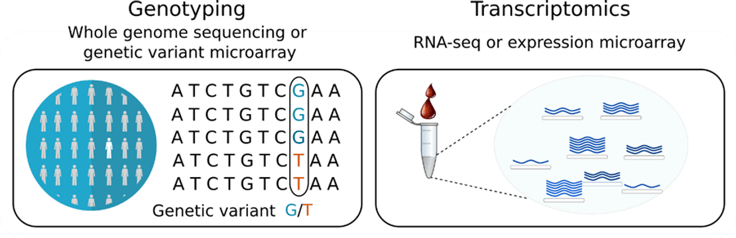
RNA-seq was used to investigate one fifth of the samples, whereas the remaining samples used expression microarrays (Võsa et al. 2021). To combine gene expression data from these different transcriptomic technologies, the authors used RNA-seq as the gold standard reference to calculate correlation scores for each gene with all other genes. Gene correlation profiles from the different microarrays were then correlated with profiles from RNA-seq data. This resulted in nearly 20,000 expressed genes tested for cis-eQTL or trans-eQTL associations in over 31,000 study participants.
How the large number of samples contributed to the results:
Sample size was also a problem when the eQTLGen Consortium tried to confirm their results with other smaller bulk RNA-seq eQTL data sets, such as GTex with just under 400 individuals (Võsa et al. 2021). The same issue arose in the analysis of a single-cell RNA-seq eQTL data set from over 1000 individuals, which the authors used to address concerns about cell composition in their original eQTL analyses (Võsa et al. 2021). In both cases, they could only confirm a small number of their initial results, likely due to reduced statistical power.
How novel higher-throughput transcriptomics could help in similar studies:
To address this, new technologies are available to boost sample sizes and therefore statistical power in transcriptomic studies by removing cost and hands-on-time as limiting factors. Large cohort studies reliant on transcriptomics are prohibitively expensive. Furthermore, if the eQTLGen Consortium had used the same transcriptomic method for all samples, study quality could have been improved by removing the need for independent pre-processing of each different microarray platform.
Although BRB-seq does not allow for the analysis of full-length transcripts, this is not necessary for eQTL expression analyses. BRB-seq detects the same number of genes as the ‘gold standard’ Illumina TruSeq library preparation method and is highly accurate and cost-effective. It is reliable even for low quality RNA samples which may be a key concern when processing precious human samples.
To find out more about how BRB-seq could help your study, please contact us at info@alitheagenomics.com.
References:
-
Alpern, D., Gardeux, V., Russeil, J., Mangeat, B., Meireles-Filho, A., Breysse, R., Hacker, D., Deplancke, B. 2019. BRB-seq: Ultra-affordable high-throughput transcriptomics enabled by bulk RNA barcoding and sequencing. Genome Biol, 20:71.
-
Nica, A.C. and Dermitzakis, E.T., 2013. Expression quantitative trait loci: present and future. Philosophical Transactions of the Royal Society B: Biological Sciences, 368(1620).
-
Price, A.L., Helgason, A., Thorleifsson, G., McCarroll, S.A., Kong, A. and Stefansson, K., 2011. Single-tissue and cross-tissue heritability of gene expression via identity-by-descent in related or unrelated individuals. PLoS genetics, 7(2).
-
Võsa, U., Claringbould, A., Westra, H.J., Bonder, M.J., Deelen, P., Zeng, B., Kirsten, H., Saha, A., Kreuzhuber, R., Brugge, H. and Oelen, R., 2021. Large-scale cis-and trans-eQTL analyses identify thousands of genetic loci and polygenic scores that regulate blood gene expression. Nature genetics, 53(9).
-
Westra, H.J., Peters, M.J., Esko, T., Yaghootkar, H., Schurmann, C., Kettunen, J., Christiansen, M.W., Fairfax, B.P., Schramm, K., Powell, J.E. and Zhernakova, A., 2013. Systematic identification of trans eQTLs as putative drivers of known disease associations. Nature genetics, 45(10).