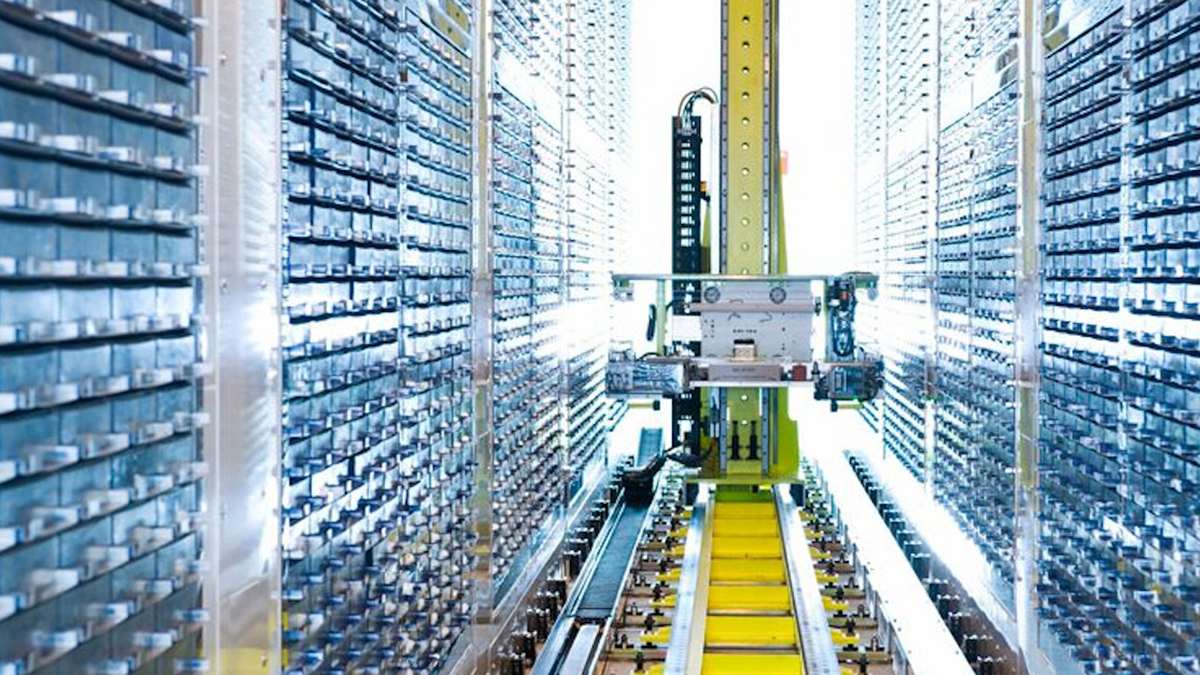
A recent ground-breaking study uses a mathematical approach to measure tumor-specific total mRNA levels from mixed tumor samples. Bulk RNA-seq data from 6,664 tumor samples and 15 different cancers show that reduced patient survival is associated with higher total mRNA levels in cancer cells (Cao et al. 2022).
In this new episode of the large-scale RNA-seq series, we look at how RNA-seq made this discovery possible and how novel sequencing technologies could help future studies.
Summary:
Many widely available cancer patient data sets provide bulk RNA-seq of tumors, often including clinical outcomes. These samples contain a mixture of both cancerous and non-cancerous cells. This mix of cells makes it difficult to use bulk RNA-seq to determine total mRNA levels specifically from diseased or healthy cells.
Alternatively, single cell RNA-seq provides a snapshot of mRNA levels from each individual cell type in a sample, but high costs make it unfeasible for large scale studies (Gulati et al. 2020).
Cao et al took a novel computational approach to evaluate total mRNA levels specifically from tumor cells using bulk RNA-seq to complement the resolution of single cell RNA-seq.
Combined with clinical outcome data, the authors show that high tumor total mRNA levels are associated with reduced patient survival, suggesting total mRNA levels as a new biomarker of treatment regimens and prognosis.
How RNA-seq was used:
Gene level bulk RNA-seq analyses usually focus on the expression of individual genes. Here, the authors aimed to calculate the sum of detectable mRNA transcripts across all genes per cell; the total mRNA level.
To do this, they refined a computational process called deconvolution. This deconvolution method separates the total mRNA levels of tumor cells from non-tumor cells in bulk RNA-seq.
To develop this, the authors first analyzed total mRNA content in single cell RNA-seq data from nearly 50,000 cells fro
m ten patients across four different cancer types. They then pooled all these cells to simulate bulk RNA-seq and saw higher tumor mRNA levels in patients with more advanced cancer and worse survival outcomes.
Next, they used this approach to investigate the 6,644 bulk RNA-seq samples from four cohorts. These cohorts included matched DNA sequencing allowing an estimation of the proportion of tumor cells and number of chromosomes per sample, leading to more accurate total mRNA levels.
How the large number of samples contributed to the results:
The largest data set contained 4,982 tumor RNA-seq samples from The Cancer Genome Atlas (TCGA) and the early-onset prostate cancer (EOPC) cohort (Gerhauser et al. 2018; Liu et al. 2018). It included samples across 15 cancer types, from colorectal cancer to lung adenocarcinoma.
In addition, they included samples from smaller cancer type specific data sets such as 1,546 samples from breast cancer patients in the METABRIC study profiled by RNA expression array, and 116 multi-region tumor samples from the TRACERx lung cancer study (Curtis et al. 2012; Jamal-Hanjani et al. 2017).
The combined use of multiple studies with large numbers of bulk RNA-seq samples resulted in high statistical power to detect small but important differences in tumor total mRNA levels.
Were it not for the large number of samples in these data sets, important prognostic features would have been missed. For example, the breast cancer cohorts from TCGA and METABRIC showed that early-stage patients treated with chemotherapy had improved outcomes when total tumor mRNA levels were higher. In contrast, early-stage patients with lower total mRNA levels benefited less from chemotherapy.
Because different treatment regimens exist for early- and late-stage cancers, total mRNA levels could be useful in predicting both prognosis and response to treatment.
The use of multiple studies with large sample numbers also allowed extensive cross validation of findings with high statistical power, removing concerns about bias from any one data set.
How novel higher-throughput transcriptomics could help in similar studies:
These potentially prognostic findings should next be confirmed with larger prospective trials, however large cohort studies reliant on transcriptomics are prohibitively expensive. Novel 3’ mRNA-seq technologies such as Bulk RNA Barcoding and sequencing (BRB-seq) address these cost and throughput issues. By barcoding and early multiplexing hundreds of samples, only one subsequent library preparation is needed.
It would enable a prospective clinical trial of thousands of samples to be performed at a fraction of the cost, while reducing both technical variation and turnaround times (Alpern et al. 2019).
Importantly, BRB-seq is reliable even for low quality RNA; a key concern when processing precious human samples. BRB-seq libraries are no different to any other bulk RNA-seq libraries and can be analyzed with the same bioinformatic packages.
To find out more about RNA-seq and BRB-seq please contact us at info@alitheagenomics.com.
References:
- Alpern, D., Gardeux, V., Russeil, J., Mangeat, B., Meireles-Filho, A.C., Breysse, R., Hacker, D. and Deplancke, B., 2019. BRB-seq: ultra-affordable high-throughput transcriptomics enabled by bulk RNA barcoding and sequencing. Genome biology, 20(1), pp.1-15.
- Cao, S., Wang, J.R., Ji, S., Yang, P., Dai, Y., Guo, S., Montierth, M.D., Shen, J.P., Zhao, X., Chen, J. and Lee, J.J., 2022. Estimation of tumor cell total mRNA expression in 15 cancer types predicts disease progression. Nature Biotechnology, pp.1-10.
- Curtis, C., Shah, S.P., Chin, S.F., Turashvili, G., Rueda, O.M., Dunning, M.J., Speed, D., Lynch, A.G., Samarajiwa, S., Yuan, Y. and Gräf, S., 2012. The genomic and transcriptomic architecture of 2,000 breast tumours reveals novel subgroups. Nature, 486(7403), pp.346-352.
- Gerhauser, C., Favero, F., Risch, T., Simon, R., Feuerbach, L., Assenov, Y., Heckmann, D., Sidiropoulos, N., Waszak, S.M., Hübschmann, D. and Urbanucci, A., 2018. Molecular evolution of early-onset prostate cancer identifies molecular risk markers and clinical trajectories. Cancer Cell, 34(6), pp.996-1011.
- Gulati, G.S., Sikandar, S.S., Wesche, D.J., Manjunath, A., Bharadwaj, A., Berger, M.J., Ilagan, F., Kuo, A.H., Hsieh, R.W., Cai, S. and Zabala, M., 2020. Single-cell transcriptional diversity is a hallmark of developmental potential. Science, 367(6476), pp.405-411.
- Jamal-Hanjani, M., Wilson, G.A., McGranahan, N., Birkbak, N.J., Watkins, T.B., Veeriah, S., Shafi, S., Johnson, D.H., Mitter, R., Rosenthal, R. and Salm, M., 2017. Tracking the evolution of non–small-cell lung cancer. New England Journal of Medicine, 376(22), pp.2109-2121
- Liu, J., Lichtenberg, T., Hoadley, K.A., Poisson, L.M., Lazar, A.J., Cherniack, A.D., Kovatich, A.J., Benz, C.C., Levine, D.A., Lee, A.V. and Omberg, L., 2018. An integrated TCGA pan-cancer clinical data resource to drive high-quality survival outcome analytics. Cell, 173(2), pp.400-416.